BKAI-IGH NeoPolyp-Small is a public dataset released by BKAI, Hanoi University of Science and Technology incorporation with Institute of Gastroenterology and Hepatology (IGH), Vietnam. The dataset has been published on Kaggle: https://www.kaggle.com/c/bkai-igh-neopolyp/
In polyp segmentation, given an input image, we need to output a binary mask where each pixel’s value is either 1 (the pixel is part of a polyp) or 0 (the pixel is part of the background). The task related to this dataset is an expansion of polyp segmentation, focusing more on the fine-grained classification to detect neoplasm polyps. In this extended task, we need to solve both the polyp segmentation and neoplasm detection (PSND) subtasks at the same time, where each pixel in the segmentation mask to have one of the three following values:
- 0 if the pixel is part of the image background (denoted by black color);
- 1 if the pixel is part of a non-neoplastic polyp (denoted by green color);
- 2 if the pixel is part of a neoplastic polyp (denoted by red color).
This dataset contains 1200 images (1000 WLI images and 200 FICE images). The training set consists of 1000 images, and the test set consists of 200 images. All polyps are classified into neoplastic or non-neoplastic classes denoted by red and green colors, respectively.
A larger dataset called NeoPolyp-Large contains about 7500 images of four different color modes (WLI, BLI, LCI, FICE) with fire-grained annotations. In the NeoPolyp-Large dataset, we also have another class called “undefined” polyp denoted by yellow color. These are highly difficult polyps where trained physicians are unsure of the classification.
All the images were collected in IGH. Annotations (including segmentation and classification) are added by five endoscopists and then are verified by two experienced endoscopists from IGH.
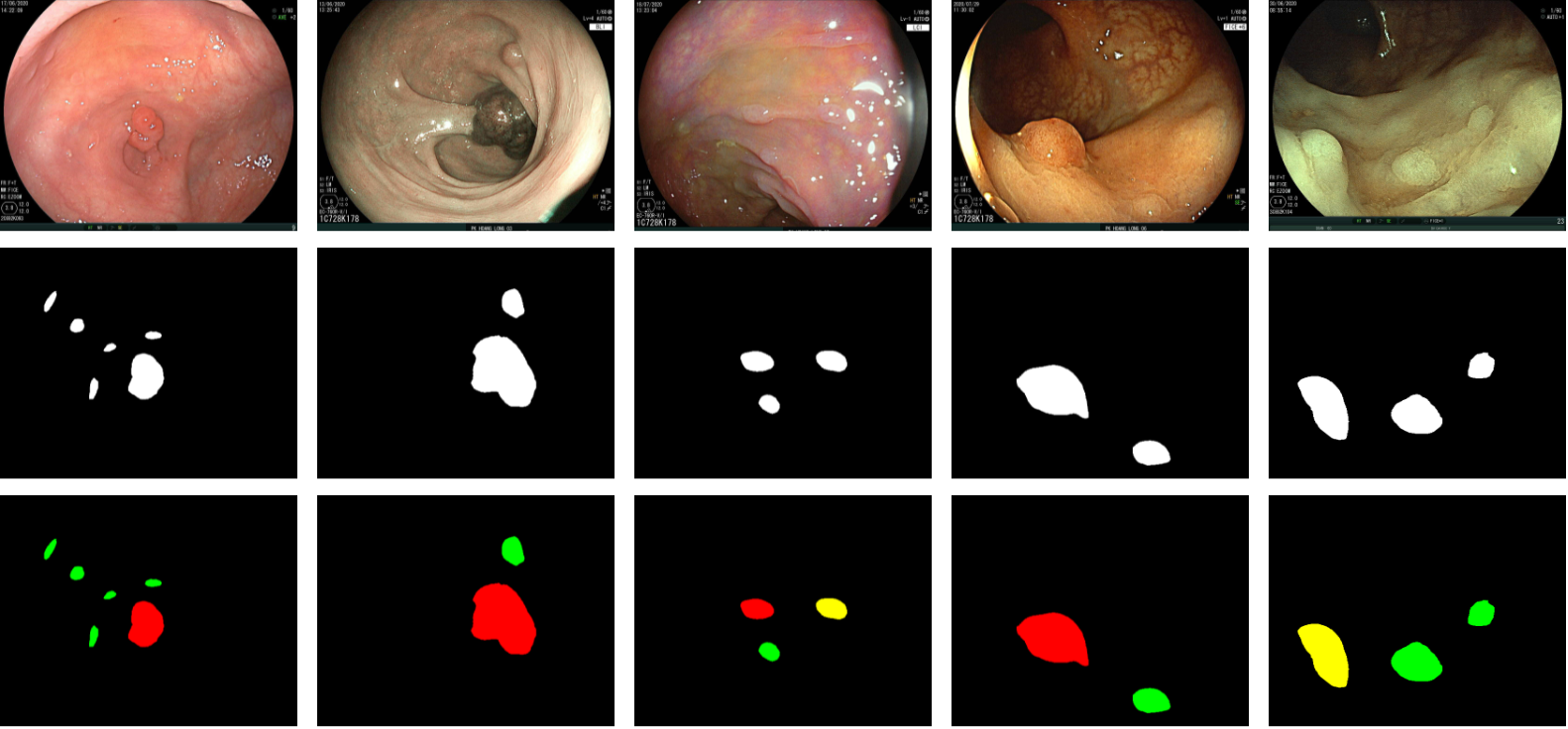
Acknowledgments
This dataset is collected thanks to the project VINIF.2020.DA17 funded by Vingroup Innovation Foundation. We thank IGH for collecting and annotating the data.
References
If you use this dataset in your work, please consider to cite the following papers:
1. Lan, P.N., An, N.S., Hang, D.V., Long, D.V., Trung, T.Q., Thuy, N.T., Sang, D.V.: NeoUnet: Towards accurate colon polyp segmentation and neoplasm detection. In: Proceedings of the 16th International Symposium on Visual Computing (2021)
2. Nguyen Thanh Duc, Nguyen Thi Oanh, Nguyen Thi Thuy, Tran Minh Triet, Dinh Viet Sang. ColonFormer: An Efficient Transformer Based Method for Colon Polyp Segmentation. IEEE Access, vol. 10, pp. 80575-80586, 2022
3. Nguyen Hoang Thuan, Nguyen Thi Oanh, Nguyen Thi Thuy, Perry Stuart, Dinh Viet Sang (2023). RaBiT: An Efficient Transformer using Bidirectional Feature Pyramid Network with Reverse Attention for Colon Polyp Segmentation. arXiv preprint arXiv:2307.06420.
4. Nguyen Sy An, Phan Ngoc Lan, Dao Viet Hang, Dao Van Long, Tran Quang Trung, Nguyen Thi Thuy, Dinh Viet Sang. BlazeNeo: Blazing fast polyp segmentation and neoplasm detection. IEEE Access, Vol. 10, 2022.