Topic:Efficient universal estimators for symmetric property estimation
Time: 11h00, Monday, September 12th, 2022
Speaker: Kiran Shiragur, Final year PhD student at Stanford University
📌 Location: Room 404, B1 Building, Hanoi University of Science and Technology
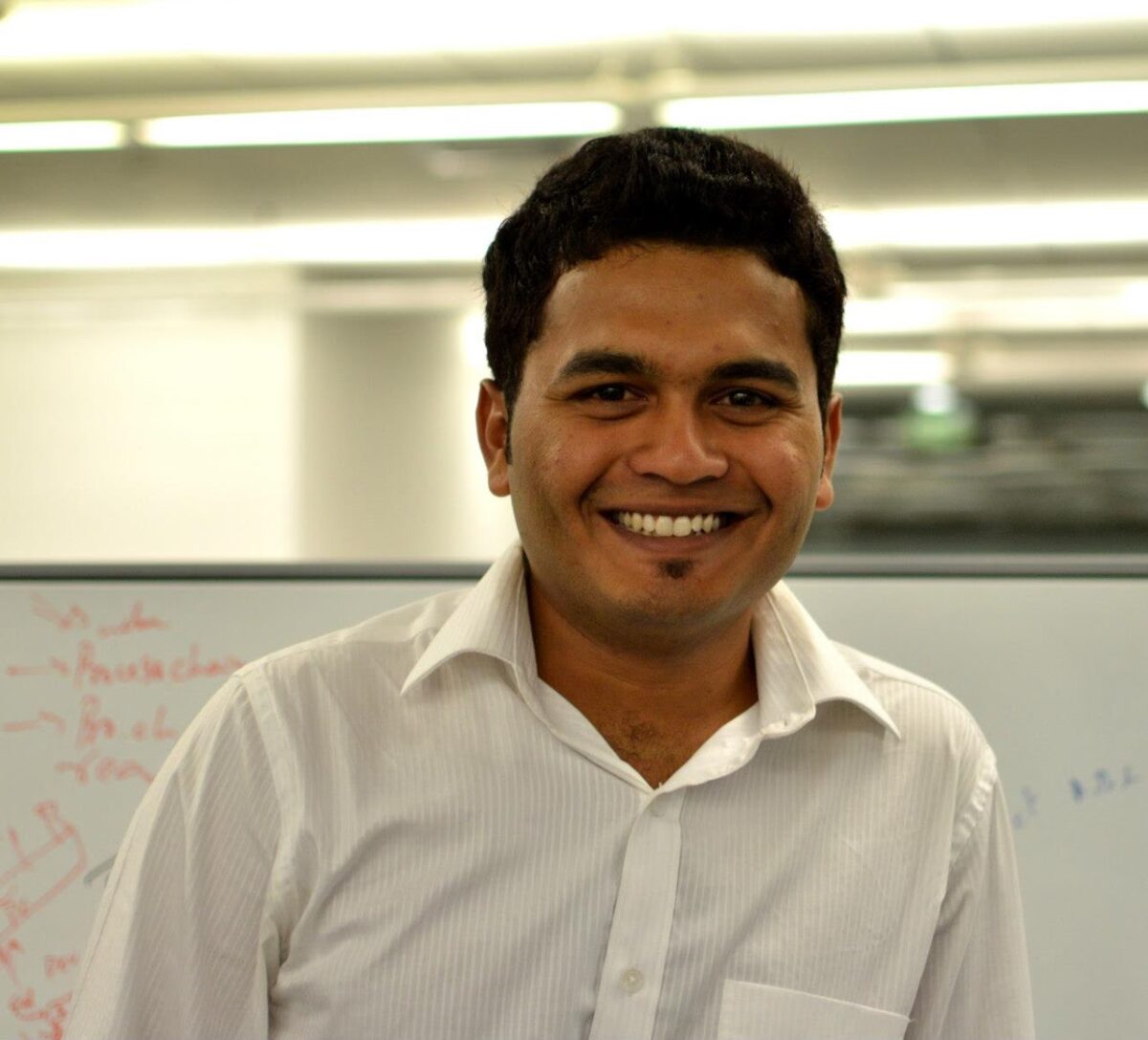
✅ Abstract:
Given i.i.d samples from an unknown distribution, estimating its symmetric properties is a classical problem in information theory, statistics, operations research, and computer science. Symmetric properties are those that are invariant to label permutations and include popular functionals such as entropy and support size. Over the past decade, this question has received great attention leading to computationally efficient and sample optimal estimators for various symmetric properties. Most of these estimators were property specific and the design of a single estimator that is sample optimal for any symmetric property remained a central open problem in the area. In a seminal result, Acharya et. al. showed that computing an approximate profile maximum likelihood (PML), a distribution that maximizes the likelihood of the observed multiset of frequencies, allows statistically optimal estimation of any symmetric property. However, since its introduction by Orlitsky et. al. in 2004, efficient computation of an approximate PML remained a well-known open problem. In our work, we resolved this question by designing the first efficient algorithm for computing an approximate PML distribution. In addition, our investigations have led to a deeper understanding of various computational and statistical aspects of PML and universal estimators. En route to achieving these results, our work has led to the design of better algorithms for deterministic permanent approximation, new rounding algorithms, faster optimization methods and novel techniques for statistical analysis.
✅ Speaker Bio:
Kiran Shiragur received his PhD from Stanford University, co-advised by Prof. Moses Charikar and Prof. Aaron Sidford. His research interests lie in the intersection of theoretical computer science, information theory and optimization. A major theme of his work involves building efficient algorithms for extracting information from limited data. Complementary to this theme, he also enjoys formulating new mathematical models and practical solutions for problems in the natural and social sciences, that lie beyond the range of traditional machinery.
Slides: HUST_slides
Recording: to be updated …