Topic: Variable selection with theoretical guarantees on high-dimensional data
Time: 15h00-17h00, Thursday, November 10th, 2022
Speaker: Dr Binh Nguyen, Postdoctoral researcher at Telecom Paris, France
📌 Location: Room 404, B1 Building, Hanoi University of Science and Technology
or online link on MS Teams: here
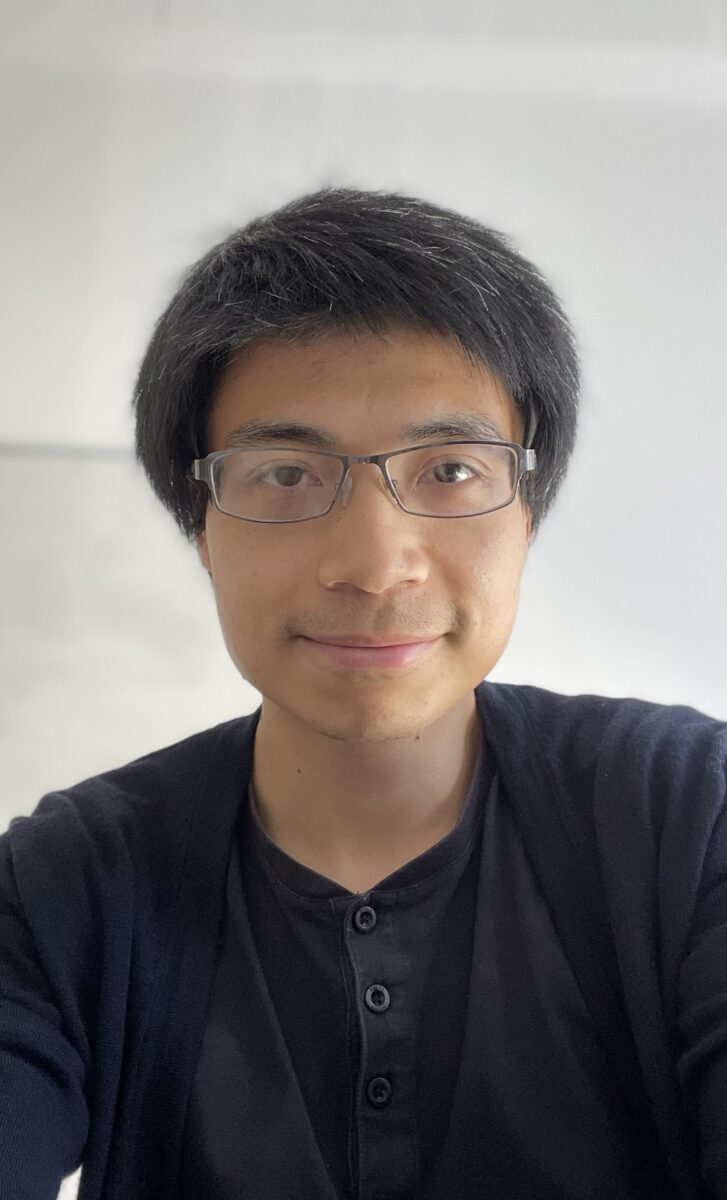
✅ Abstract:
In many scientific applications, increasingly bigger datasets are being acquired to describe more accurately biological or physical phenomena. While the dimensionality of the resulting measures has increased, the number of samples available is often limited, due to physical or financial limits. Performing statistical inference in such high-dimensional setting remains a hard problem that suffers from the curse of dimensionality. In this talk, we will first go through an introduction on the knockoff filters, a recent advance in multivariate analysis that controls the False Discovery Rate (FDR) with limited distribution assumptions. We then present a method for aggregating several samplings to address knockoff filter’s randomness, one of the its major limitation. We provide non-asymptotic theoretical results on the
aggregated knockoff, specifically guaranteed FDR control, which relies on usage of concentration inequalities. Furthermore, we extend the method, providing a version that can scale to extremely high dimensional regime. One of the key steps is to use randomized clustering to reduce the dimension to avoid the curse of dimensionality, and then to ensemble several runs to tame the bias from the selection of a fixed clustering. We show that our algorithms perform reasonably well in practical applications from life-sciences, such as neuroscience, medical imaging and genomics.
✅ Speaker Bio:
Binh Nguyen is a postdoctoral researcher at Telecom Paris, France. He obtained his doctoral degree in statistics in Département de Mathématiques d’Orsay and INRIA, and a master degree in Data Science at Paris-Saclay University. His research interest are in high-dimension statistics, density estimation, and more recently the application of optimal transport to structured prediction problems in machine learning.
Slides: to be updated…
Recording: to be updated…